Last Updated on August 14, 2022
Interview with Dr. Ted Hayduk
Dr. Ted Hayduk sat down with John from Sports Degrees Online and discussed Dr. Hayduk's unconventional entry into the sports industry, some misconceptions about sport analytics, and some interesting behind the scenes info about how sports organizations use data to their advantage.
About Dr. Ted Hayduk
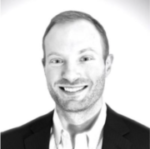
New York University
Ted Hayduk is an Assistant Professor at NYU. He does academic research focuses on technology startups in sport and entertainment. His work has been published in a number of leading journals, including Journal of Sport Management, Journal of Sport Economics, Sport Marketing Quarterly, and International Journal of Entrepreneurship and Management.
Interview
Can you give us some background on your career and how you have worked to get to this point?
I got started in the sports industry haphazardly on the consulting side. The boutique firm I worked for mostly focused on the operations and security of critical infrastructure, such as railroads, national monuments, and government buildings; anything you would consider critical to international and national transportation of goods and people. Right after the Boston Marathon bombing, a lot of the sports leagues started to think about how they could revamp things on the operations side by bringing safety and security to the forefront. That was what our team was tasked to do.
We went around to the Major League Baseball stadiums and conducted research, looking at things in the legal environment, the org’s business operations and strategy, and their use of tech. We put together recommendations for best practices based on where they were versus where they probably needed to be. A lot of times that required the implementation of new technologies and operations protocols. Once that contract was done, I found myself really interested in gathering information and doing research as a neutral third party and educating people about what I found.
After some conversations with the firm and some of my old professors from grad school, I kind of realized being a research professor at a university is basically the same thing. That was the impetus for getting a PHD. It helped me add the academic rigor and quantitative skill sets to the research base that I had built up. That’s how I found myself in academia. I love NYU specifically because we are expected to do the academic research and be active on the publishing side, but we’re also expected to keep one foot in the industry and interact with business managers and start-up founders and do research with those entities. It’s very applied, practical, and consultative.
I wonder, doing that analysis of the older stadiums like Fenway and Wrigley, were those harder problems to solve? If you think about it, they were built a hundred years ago and there was no way they could have foreseen what the world was going to look like in the future.
Yes, a lot of those were a challenge, especially from a physical space perspective. The older ones tended to be in urban locations downtown. You think about security in terms of concentric circles where ideally you want to push out as far as you can, but in urban settings that’s really difficult to do.
Are there any sports analytics myths or misconceptions that you would like to clear up? I’m curious if you think movies like Moneyball have helped or hurt the accuracy of peoples’ perception of sports analytics?
I think we are ready for another iteration of Moneyball. There have been some really good books since Moneyball that accurately capture the state of analytics in sports business. One of the popular myths is that sports analytics means analysing game outcomes, engineering runs or scores or whatever. But in reality, for every analyst looking at ‘on field’ outcomes, there are probably three to five analysts in the business strategy or marketing verticals. And, generally speaking, these two groups don’t speak to each other because they are concerned with very different outcomes.
“One of the popular myths is that sports analytics means analyzing game outcomes, engineering runs or scores or whatever. But in reality, for every analyst looking at ‘on field’ outcomes, there are probably three to five analysts in the business strategy or marketing verticals. And, generally speaking, these two groups don’t speak to each other because they are concerned with very different outcomes.”
The other myth is that analytics is a remedy for winning– “If you implement these methods you’re going to make the playoffs, have a hundred percent capacity and go on to do these great things.” In reality, in addition to having the competency and the staff behind analytics, you also have to have the facilitating mechanisms, such as coaches, GMs, and owners, who are in alignment and who are devoted to a data-driven strategy for doing business. You could have the most advanced models telling you the best way to play against San Antonio’s offense is to guard in a certain formation, but if the coach says, “No thank you”, then it’s a moot point.
“You could have the most advanced models telling you the best way to play against San Antonio’s offense is to guard in a certain formation, but if the coach says, “No thank you”, then it’s a moot point.”
By contrast on the business side, it’s a much easier argument to make. They might say, “If we just tweaked this one aspect of our app interface, it’s going to increase engagement by four percent.” Here you can very clearly tie those things to revenue.
That makes a lot of sense. It seems like you could certainly come from another analytics industry and jump into sports analytics. It doesn’t seem like you need to be a big sports fan to be successful in it.
That’s right. This week I saw a really great post by a start-up out of Austin Texas that’s essentially providing an outsourcing option for clubs that are maybe a little deficient in their analytics department. They focus on “on the court outcomes” so they aren’t so much on the business side. But this is a really tough competency to build out and develop into a competitive advantage.
It’s a lot of money and time learning about an area that’s still kind of new. So they see it as their value proposition to provide this to teams as a service. I don’t think any of those folks that are out of Austin would be described as die-hard sports fans. They did their PhD’s in spatial temporal modeling – how things move through space. It’s great if you want to model how flocks of birds migrate each year or how schools of fish move together, and now they’re taking those skills and applying them to the sports setting. So, while knowing the nuts and bolts of a sport’s strategy helps a lot – it’s not the central characteristic of an analyst that makes her so valuable. It’s the ability to take models from other areas of science and apply them to a sports setting.
How important is mathematics for someone looking to get into sports analytics? If someone is not strong in math, does that mean this isn’t a great career choice for them?
Good question. There is a subtle difference between math and statistics which is worth pointing out. Statistics is an applied math. In an ‘pure’ math, you’re doing a lot of rote calculations and memorization. This is probably the math people have nightmares about, writing long proofs without learning about the real world application. That was always my hang up, wondering what it was going to help me do. Even if you think of yourself as someone not great at math or not super comfortable with math, I would encourage you to take a statistics course.
A lot of the models in analytics are really just middle school applied math like linear algebra and matrix algebra, but on steroids.
What sort of jobs or positions are out there for young people who have an interest, and have studied sport analytics. And, do most positions require a degree in some kind of statistical analysis, or are there opportunities for people who are self-taught?
Let’s talk about the second one first. There are plenty of opportunities for self taught folks to get into this space. I was keeping a running tally of all the remote and digital opportunities to learn these things but I’ve stopped keeping track in the upwards of twenty. Coursera for example is a great way to get involved or at least scratch the surface. It’s forty bucks a month. If you’re curious, it’s a great way to get started. DataCamp is another great one. Our group at NYU is offering a Certificate program in Sports Analytics starting this fall. Increasingly, universities are offering versions of their classes in a recorded format on YouTube. There are all kinds of opportunities to pick up these skills to gain your way in. If you want to go that route, the biggest thing you can do is conduct your own analyses in R or Python and keep a blog detailing everything you do. It’s a way to show teams and leagues that you really know your stuff.
It certainly helps to have a quantitative background in terms of education. If you have an undergrad in general business along with some kind of focus in statistics or math, that would lend you well. Many sport management programs are also offering concentrations in sports analytics or sports tech, as we do here at NYU.
I’ve had some discussions with students that were interviewing to work in the analytics department of some major sport franchises. This process is becoming pretty similar to the interviews you would expect at the big consulting firms, or at Google or Facebook. Increasingly, they don’t want to know everything about you from a piece of paper. The ability to concisely break down a big problem into a series of logical steps consisting of smaller problems at each step is just as valuable as an undergrad in statistics.
The types of jobs we are seeing, they are calling them “analysts” which is a pretty generic term for someone that is going to come in with a a pallet of skill sets, where they can do three to five things pretty competently. The organization can then plug them into projects that are ongoing. Part of that skill set is pulling data from a database. A lot of teams now have their own bespoke data lakes and clouds that they are requesting fields of data from. Teams need to pull, clean, analyze, and break down data to build working models that are also high-performing.
Then, communicating those results is a big part of an analyst’s job. You have to sell the idea up the hierarchy. No one will listen to you if you say, “We should do x because that’s what the numbers say.” There is an element of salesmanship, communicating the results, iterating to get feedback from your colleagues, and then revising the models. It’s a consensus building process. For example, evolving the NBA from a two-point to a three-point league didn’t happen overnight. It was a gradual process that took a few years.
“Then, communicating those results is a big part of an analyst’s job. You have to sell the idea up the hierarchy. No one will listen to you if you say, “We should do x because that’s what the numbers say.” There is an element of salesmanship, communicating the results, iterating to get feedback from your colleagues, and then revising the models. It’s a consensus building process.”
How do you see the field of sport analytics changing in the next five to ten years?
As I mentioned before, the interview process is increasingly becoming like that of Google or Facebook. I think it will become more like that. There is an interesting overlap of ownership between tech and entertainment. If you look at the ownership of sports franchises, they bring that tech background with them. Steve Ballmer who was Co-Founder at Microsoft all those years ago and helped build it into everything that it is today, he’s taking Microsoft HR processes, evaluations and blueprints of how that company used to run day to day and bringing it to the sports world. You could say the same for Mark Cuban or Ryan Smith. I think it’s going to get increasingly rigorous and it may get increasingly competitive because there are a finite number of roles to be filled.
I also think we’ll start to see a quantitative shift into other parts of the business. The Miami Heat do that really well. They subject almost every other part of their business to a very rigorous academic skill set in terms of validating ideas and pursuing certain opportunities. I think it will become more institutionalized. Folks will start to have more data science skill sets rather than statistics. Data science is a ‘full stack’ skillset, whereas statistics is more specific to the model building and validating stage. New analysts will need to acquire data, clean data, manipulate data, generate new data from old data, build models, test and train those models and then actually implement the findings. It’s a whole range of skill sets that will be increasingly sought after.
“Folks will start to have more data science skill sets rather than statistics. Data science is a ‘full stack’ skillset, whereas statistics is more specific to the model building and validating stage. New analysts will need to acquire data, clean data, manipulate data, generate new data from old data, build models, test and train those models and then actually implement the findings. It’s a whole range of skill sets that will be increasingly sought after.”
Learn more about Sports Analytics Careers
I can see how data scientists will be more valuable. As time goes on, the tech industry keeps finding new ways of aggregating data. It’s so much to handle really.
And it’s all around us. When we are in the stadium now, we are giving off data all the time. The NFL announced they are going one hundred percent mobile ticketing. They can argue it’s more secure and seamless; It gets people in and out more efficiently. It’s also a gateway to getting inside their mobile devices. If you have to download their app to utilize their digital tickets, now they can interface with all the other data consumers are giving off. If you are purchasing team gear on Fanatics, scrolling through Instagram and interacting with team content, they’ll be able to package that into one big user profile.
What are some interesting projects you have seen recently in the world of sports analytics?
I always find myself most interested in consumer behavior. Because the economics of consumer decision making is really interesting to me, particularly the economics of sport fan decision making versus consumers in other industries. We know that sports fans are a little irrational sometimes, there is a little bit more of an inelastic demand with respect to prices they are going to pay for tickets, merchandise, etc. Understanding why and how that happens is interesting.
I was recently lucky enough to get brought into a project in partnership with two NFL teams to do an experiment consisting of an app interface and a ticket buying experience. They were interested in partition pricing, which we see all the time on Ticketmaster. Typically you are going to get a ticket price plus some type of processing fee that’s going to make you angry, then some total. How they present those numbers to you psychologically impacts your willingness to pay.
In this experiment we found out you can actually increase the demand and willingness to pay for tickets if you attach mandatory fees to causes that are related to environment sustainability or community engagement. Even if you are physically paying more for a ticket and you don’t actually get anything additional for this mandatory fee, if this fee goes to something that is perceived as socially beneficial then it actually increases the demand and willingness to pay for that same exact product. If you tie that mandatory fee to something that’s not useful or too profit-oriented, demand wanes.
I think there are a lot of clear implications with respect to pricing of tickets and creating value at the same time. It creates a win-win-win scenario for consumers, teams, and social causes.
And if you can make the buying process more enjoyable or less painful, chances are they are going to be a repeat customer. I’m playing out the scenario inside my head, and if I was paying for something with a fee and no explanation, my first thought is to look for another app or way to do it. But if part of it is to do some carbon offset or partner charity, it makes me feel better about my purchase.
Exactly. And, even more interesting is that we recreated the experiment in four or five different ways and got the same result – whether the cause was directly under the team’s umbrella, or whether it was attached to a parent brand (i.e., the NFL). Even if it was a nonexistent cause, demand was still elevated. You can create extra value to your product when you attach them to those types of causes. It’s really interesting from the analytics side–the consumer behavior and pricing perspective.
Sport Analytics seems like a pretty new course offering for colleges and universities. How long has NYU offered its sport analytics course, and was there a sort of impetus for offering the course?
The analytics course or suite of analytics courses we offer are relatively new. I’ve just finished up my second year at NYU, but these courses predate me. But the foundational type of courses that we offer which we call ‘Foundations of Sports Analytics’ are iterations of Research Methods courses that we’ve taught for a long time. So it’s always been a part of our program.
I think that speaks to the trend that’s dominating as well. If you’re trying to teach a class to students called ‘Research Methods in Business’, it’s going to be tough to get them engaged. And that makes sense because R&D wasn’t traditionally a big deal in sports and entertainment. But now, with this adoption of analytics, that’s essentially what being a data scientist is — doing quantitative R&D. When you frame it like that to reflect an all-inclusive approach, that’s where you start to get students more engaged.
We have some more new courses like ‘Basketball Analytics’ and ‘Gridiron Analytics’. These have just started to be offered in the last five years or so, but the other ones are just slight tweaks and updates to classes we’ve always had.
Visit our Master’s in Sports Analytics Guide for complete information about programs currently being offered.
What advice would you give for someone who is looking to go into the field of sport analytics?
I get a lot of students who are intimidated by the quantitative aspect of analytics. They say, “I wasn’t great at math so I’m just anxious about how I’m going to do in the master’s level course offerings since there is a required analytics course’. There is some sense of apprehension. My advice would be not to let that discourage you. More important than knowing the technicalities of the model building process and the econometrics that underlie it, is that it’s important for folks to understand conceptually what’s going on. And, there is a lot you can learn at this level. It’s not just sitting in a room and doing math on a white board. It’s learning about tech integration, presenting new ideas and data science skill sets as referenced earlier. If there is a little voice in your head that is making you interested but apprehensive, just dive in anyways because there is so much out there.
“It’s not just sitting in a room and doing math on a white board. It’s learning about tech integration, presenting new ideas and data science skill sets as referenced earlier. If there is a little voice in your head that is making you interested but apprehensive, just dive in anyways because there is so much out there.”
Last question here, what are some good books, podcasts, or other resources for people looking to learn more about sports analytics?
There are a couple books that are kind of neutral and apply to all areas of business and then there are some that are a little bit more targeted. One of the best iterations of Moneyball is called Beta Ball. It’s about the Golden State Warriors and how they have taken a tech mindset to running the team. We have seen the fruits of those labors over the past ten to twelve years. They went from a perennial underdog to a super dominant team pretty quick. They are in the thick of it now. That book is really good.
Another one is called Algorithms to Live By: The Computer Science of Human Decisions. That was written by two former Google managers. That’s really interesting because it talks about the cognitive biases that we all have when it comes to making decisions in life and how we can apply those to other areas of business or personal relationships. That one’s interesting because it spends some time talking about what exactly algorithms are, and removes that veil of mystery. Algorithms are really just a set of rules used when you perform a task. I really like that one.
Another one is, The Man Who Solved the Market. I recommend this a lot to my students who are interested in the finance and economics side of sports business because this is a book that chronicles the quantification of the finance industry beginning in the mid 70s. It’s crazy to think about it but the finance industry was not particularly quantitative back in the day. A lot of investment decisions got made on gut instinct. There was a guy named Jim Simmons who came along and spearheaded this whole quantification trend. This book chronicles their growth over the past twenty-five to thirty five years, and when I see what’s going in the sport industry, I can’t help but draw a lot of parallels.
There is another one called A Master Algorithm which I think is pretty cool. Pedro Domingos wrote that one. He talks about these different areas of machine learning and artificial intelligence. There are four or five main pillars of this field and he talks about how to integrate all these into an algorithm that would be as close theoretically to human capabilities as we could get at this point. Again, he takes the veil back and looks at AI and ML, defines what they are, what their benefits are and what their weaknesses are.
In terms of tools, R a great entry level coding software, and the publish free e-books about certain topics. One of my favorites is Econometrics with R. R is completely free; which is even better. There are a million and one free resources out there and I am seeing more and more books out there on the topic too. It’s great if you are a self-learner. You don’t need crazy expensive software or expensive textbooks to get involved.