Last Updated on June 9, 2022
Interview with Professor Erin Schliep
Sports Degrees Online recently chatted with Professor Erin Schliep from the University of Missouri. They talked about the the role of data in sports, how MLB teams use data to maximize player and team performance, the growth of the sports analytics field, and potential roles available for graduates.
About Professor Erin Schliep
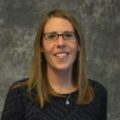
University of Missouri
Dr. Erin Schliep is a Professor of Statistics at the University of Missouri who completed her PhD at Colorado State University and was a postdoctoral fellow at Duke University. Professor Schliep's research interests include statistical methodology for multivariate, dependent data including spatial and spatio-temporal modeling.
Interview
Bryan: Can you tell us a bit about your journey into the field of data and sports analytics, and the trajectory that has brought you to this point in your career?
Prof. Schliep: I started an undergraduate degree in mathematics and accounting because I had a love of numbers. I was a Division III golfer while in college. I was always passionate about sports, but I was particularly interested from the numbers and analytics perspective. I went to graduate school for statistics to further that degree and area of interest. When I was in graduate school my intention was to go back and teach at a liberal arts school post getting my PhD because I loved the aspect of teaching and interacting with college age students.
While in graduate school, I fell in love with research. Working on research projects, particularly collaborative research projects, motivated me to continue both research and teaching. And that’s what got me here to the University of Missouri. A lot of my current research interests have stemmed from collaborative research projects. The best part about being a statistician is that we can work in nearly any application area we want because statisticians are in demand in areas of research whether it’s medicine, economics, or social sciences. A lot of the collaborations that I established in graduate school were in environmental science and ecology. I’ve built up quite a few collaborations starting from graduate school, continuing now to my sixth year being a professor. That is the trajectory so far.
Recently, I have started thinking about the connections between environmental and ecological statistics and sports. For example, when we think about how animals move across a landscape or how events occur across space, you can start to think about the connection between that and how sports, athletes and games are played. There is an immediate connection with some of the methods we create for tackling challenging problems in ecology and how they can directly relate to sports. The way animals move across the field may be similar to the way soccer players interact across a soccer field, so there are a lot of interesting areas ripe for research that span these two areas. That has made this natural connection between thinking about the statistics for ecology and the statistics for sports.
I was always passionate about sports, but I was particularly interested from the numbers and analytics perspective.
Bryan: Please tell me more about some of the specific research projects that you’ve been a part of.
Prof. Schliep: I spent some time working at Duke University where I worked with some researchers in forestry and ecology and thinking about, for example trees – if we look at the way they are located across a particular forest, do we see any sort of negative dependence between their locations and the size of the tree? Thinking about this in the statistics world, in particular the spatial statistics world, the random set of locations of trees is known as a point pattern. Given the locations in space, can we identify some dependencies between where objects are being seen? This is one of the things you think about in forests because you imagine that big trees don’t necessarily occur close to other big trees – they are fighting for space or fighting for resources across a forest.
That’s one particular project I’ve been excited about working on. More recently, I’m involved in a really large collaborative research project focused on studying lakes across the United States, particularly important features of those lakes. Nitrogen and phosphorus are often measured in lakes and can have some really important impacts on the ecosystems within the lakes. One of the big parts of this project is the data collection process where we are gathering data from a bunch of different state and federal agencies, schools, or private stakeholders. Our goal is to try and build this big data repository that has information about all the lakes across the entire United States.
You can imagine not all lakes are being sampled – maybe not every year or not sampled at all. It’s our job as statisticians to figure out a way to make predictions at locations that we don’t go. We try to identify information in the data collected that can help us make informed predictions for locations we are unable to observe.
One of the big parts of our work is to build statistical models. The focus of statistics is not only about making predictions but also capturing and quantifying uncertainty. We aim to make a prediction about a certain quantity or measurement of interest that we don’t observe and give some indication or measure of how good that estimate is going to be.
The interesting perspective I can bring to these collaborative projects is the statistical or methodological experience to address these types of questions. The questions themselves are usually driven by the ecologists and environmental scientists on the project.
Bryan: As someone who is confident in statistics and analytics, do you get excited about looking at vastly different things, knowing that you are going to be able to shed some light on data sets? How do you leap around from something like ecological patterns to something like sports for example?
Prof. Schliep: From a statistician’s perspective, you get excited about challenging problems. Whether that means the data set has a lot of noise or measurement error, or there are a lot of observations where we are trying to understand how we are going to leverage all of the information.
You hear this coin, “Big Data” which has been thrown around for the past decade. From a statistician’s perspective, there are a lot of challenges to gain as much information from a data set as you possibly can. Our objective is to find information and tell a story from the data. That’s the job of the statistician, to come up with ways to do that.
Oftentimes when I see an interesting data set, I put it in my back pocket and say “That would be a good one for a class”. Or sometimes I say, “That would be a great one to extend a current methodology or to try and push the envelope a little further in a particular application area.”
Most applied statisticians that you talk to are motivated by the application. So, if someone knocks on my door and gives me a data set on baseball pitches or strike counts throughout a particular season or player, we might try to see if there is a difference between where our pitchers are throwing the ball when the count is 0-0 versus when it’s 3-2. If so, and you are the batting coach for the other team, you want information on how this individual tends to throw the pitch for the given count. Anytime you could answer that and put it into practice, that’s exciting and that is why I do what I do.
Bryan: Very interesting. So how is sports analytics different from other types of work you’ve done and how is it similar?
Prof. Schliep: One of the biggest challenges for sports analytics relative to some of the other fields like ecology and forestry is the issue of getting access to real data. Usually in ecology and forestry, people are willing to share their data once they’ve published on that data. If the trees are being measured every year and you know how big they are and where they are, it’s totally open science for someone to come take that data and do something different.
Whereas in sports, there is a competitive advantage. Teams do not want to give up their data or any sort of information based on what they are collecting. I think that is probably the biggest challenge or difference between the sports field itself and other fields in terms of building statistical methods. You have to have some sort of real data in order to learn from it. You can’t just generate data for the sake of hoping to capture what is maybe happening for a particular game or sport. I’d argue that some sports are better at it than others. I know for example the SEC for baseball has made it such that information from the game is publicly available within the SEC. But different conferences and different sports are sharing data in different manners or maybe not at all.
Bryan: As an example, let me ask about data in MLB – are teams buying data from agencies, or do they source data from their own staff?
Prof. Schliep: It definitely varies. Speaking from what I’ve heard from people that work in MLB, it’s owned by the team. They have different types of sensors throughout their stadiums that are used to gather information. When the ball is hit in a certain direction, can they figure out what speed it came off the bat, the trajectory it went, and where it landed? That’s all being captured by the devices that they have built into their stadiums. But that’s not going to be shared amongst different programs.
In addition to this type of data, another interesting aspect of sports analytics is from the sports performance side. That’s coming from the monitors the players are wearing themselves. It’s become very common now in professional sports and also in the collegiate level that players have GPS monitors on them. Football, hockey, soccer players, even at the collegiate level, have these GPS trackers and heart rate monitors and different measuring devices that produce very high resolution data. You can get twenty observations per second, for example, on the movement and position for these individuals as they are running across the field. That’s the type of information that is again, not going to be shared.
Bryan: I can imagine that some of that data is being analyzed from an exercise science perspective, trying to maximize each athlete’s performance and how it relates to everything else.
Prof. Schliep: You need to maximize each individual’s performance and maximize their collective behavior. If you’ve got five basketball players on the court, you want each person to be playing at their best and you want those five people navigating the court collectively at their best. There are a lot of moving parts here to be thinking about how you can optimize this. You have the strength and performance coaches trying to make sure these individuals are at their best on game day. Then you have the coaches applying their tactics and strategy and making sure they are performing well collectively, as they set up their offensive or defensive positioning.
Bryan: It sounds based on what you described that analytics data just goes deeper and deeper. The more you want to look at, the more you can look at, the more it’s possible to find competitive advantages. With regard to the job market for sports analytics graduates, what types of opportunities are out there? What types of careers are emerging in this field?
Prof. Schliep: I think any time a coaching team is able to learn something from their data science team which results in a realized advantage for their team over another, it grows the sports analytics field more. We saw a few years ago, there was an analysis that studied corner kicks in soccer and identified a positive relationship between the number of corner kicks and goals scored. After that, corner kicks and set pieces became a major focus for teams throughout the league.
Anytime you see an advantage that is data-driven and obtained from someone doing an analysis, it makes the demand higher. With Covid and its economic impacts, it’s hard to know exactly how sports teams are growing their analytics departments. However, more broadly, if you look at particular baseball programs, you’ll see ten years ago they had two analysts and now they might have twenty.
“I think any time a coaching team is able to learn something from their data science team which results in a realized advantage for their team over another, it grows the sports analytics field more.”
Bryan: Wow, that’s a huge jump.
Prof. Schliep: It all started from baseball but it’s becoming much bigger across all sports. Again, it will take a little while for some sports to catch up but there are definitely some big departments within some professional sports organizations that have some sizable sports analytics groups.
Bryan: Looking specifically at those departments – the baseball team analytics department that grew from two to twenty on the baseball team, for example – can you tell me what the job looks like on a team like that? Do those jobs pay well? Would you say this is a fulfilling, fun job? Is there variety, or is it just gathering and crunching numbers?
Prof. Schliep: Good question. Because I don’t personally have that job I can only speak from my experience talking to some folks that are in that particular field. I think they find it extremely satisfying. Any time you can contribute to your team, making better decisions whether it’s from a training perspective or performance in a game, I think that’s extremely fun. The nice thing about the sports field in particular is that while much of your job is going to be sitting in front of your computer screen and crunching out algorithms and models to tease out those stories from the data, you will also get the opportunity to present the data and results to those making actionable decisions. You have to be able to communicate not only in statistics but with coaches and general managers about the analysis that you did. All aspects of the job are important, ranging from doing the statistical analysis to creating understandable visualizations and communicating results.
Bryan: Looking at the next ten years for example, would you say you agree this is a growing field? Is sports analytics a field that you would recommend getting into?
Prof. Schliep: It has to be growing and exploding. Just in the way data collection and the technology is advancing. We see it now. I’m a runner and it’s so fun to look at my Garmin or Apple watch after a run to see summary measures of my workout, goals reached, training plans, and recovery needs. Sports is a big money-making industry, and data are being collected on the teams and athletes all the time. You have to imagine that this problem is not going away and we need statistically savvy people to analyze this type of data.
“Sports is a big money-making industry, and data are being collected on the teams and athletes all the time. You have to imagine that this problem is not going away and we need statistically savvy people to analyze this type of data.”
Be sure to check out our Sports Analytics Careers Guide for more information about jobs in the field.
Bryan: Looking at your program at the University of Missouri, you offer undergraduate and graduate certificates programs. Can you tell us just a bit about each program, and what are some of the highlights?
Prof. Schliep: The undergraduate and graduate programs are very similar in terms of what they offer the students. Particularly, the undergraduate is directed towards students that are exploring the field itself, and still working on their undergraduate degree. They may or may not be statistic students. The graduate certificate is intended for students that are passionate about sports and the field of sports analytics. For them, it provides a nice opportunity for them to learn some of the statistical methods and analyses that would be useful for getting one of these jobs in one of these sports offices.
The main distinction between our program and any of the other sports analytics programs you can find across the US is that our program is currently the only one that stems from a statistics department. We are very focused on the students obtain a strong statistical foundation, so that they can do analysis, draw inference, and make predictions while accounting for uncertainty. There are many sports analytics programs outside of the US in the UK and Australia, but a lot of the programs across the US are more focused on the more business side of sports, coming from maybe economics, management, or sports performance and not focused on the statistical methodology.
Our certificates consist of four courses, two that are very quantitative and methodological – students learn the nuts and bolts of doing analyses that are statistically sound. They learn to use computer software like “R”, a computer programming language for data analyses and visualization. The other two courses are focused on how we can use these methods to answer questions of interest in sports. They spend some time in those courses interacting with folks that are working in the field and have jobs in these offices. Some of these are alumni of our program or contacts that we have across the state. Students get to hear first-hand what it is like to work in this industry and the types of questions that they might be asked to answer if they were working as a sports analyst.
For more information about related degrees, visit our Master’s in Sports Analytics Degree Guide
Bryan: I imagine that you have been in touch with some recent graduates from these programs. So if we look at the graduate program for example, are these graduates getting jobs and are they good jobs?
Prof. Schliep: Our program is brand new. We are graduating our first set of graduate certificates this spring, so I’ll be able to answer that question a little bit more clearly in the future. With that being said, we have three, maybe four recent grads from our program that either earned undergraduate degrees in statistics or master’s degrees in statistics that are working in professional baseball. The nice thing about having alumni in these positions is that they are a direct contact for our current students and reach out to us when career opportunities are available. They often send us messages when their offices are hiring and looking for another Mizzou grad. That has really provided a great benefit for our current students. They have been able to get advice from some of these folks to see what it is they should be focusing on and how they can best put themselves out there and get hired into one of these fields.
Bryan: That’s a great sign and exciting for your program. Based on what you said on the unique nature of your program and it’s focus on statistics, would you say this degree in particular, while it specializes in sports, would also be applicable in other areas if they decided they wanted to change their career and pursue a job in some other area of statistics?
Prof. Schliep: From the methodological perspective, absolutely. These courses provide the backbone of data analysis and statistical learning for our undergraduates and would serve you well in any sort of field you decided to apply your statistical and quantitative skills. The two courses that are focused more on sports analytics would provide some nice applications and projects to add to your resume showcasing research and analytics experience.
Bryan: Is there anything else that you’d like to touch on for people that are considering a career in sports analytics?
Prof. Schliep: I would just say, if you are interested in sports analytics, don’t overlook the statistical, mathematical or quantitative skills because those skills are extremely important if you are trying to get into this field. The whole field is driven by data and how you can learn, find stories and tease out information from data. In order to do that, you have to have the quantitative skills. Math, statistics, and computer sciences are all very important to building your foundation and to being successful.
One of the big components of that is learning the computer software in order to analyze data. You’re not going to be crunching this data into your TI-83 calculator, so getting comfortable with computer programming, whether using R or Python, or other computing software, is critical. My last comment is that students that have been the most successful in procuring jobs are the ones that have something to show. They have a project they have been working on, a shiny app that they’ve made, a dashboard on a website or a blog, something to show what they have done. It illustrates to the person hiring that they have real-world experience in sports analytics. Visualization skills, computing skills, statistical skills, and communication skills — all of those are extremely important to get into this career field.
Bryan: These types of projects, these shiny projects that the best candidates are able to display that result in them getting very competitive jobs, are these things that they have created on their own as passion projects, or did they create these projects as part of your program?
Prof. Schliep: Sort of both. Interesting story, one of our former Mizzou master’s students created a website as part of his master’s project called umptracker.com. You can go there and see the main screen but it’s not a working, up-to-date app as it once was. But what this app did was track umpires and the decisions umpires made in terms of calling pitches as balls or strikes throughout games. It was live and it would update every day to identify which umps were making the most right and wrong calls or best of worst called on a particular day.
The reason I mention this is that he had this website live for a couple of years while working at a job in Kansas City that was not related to baseball, and the Boston Red Sox called him out of the blue and offered him a job. Now he’s working for the Boston Red Sox in their analytics office. It’s a perfect example of having a product out there, done as a project while studying at Mizzou, that led to his dream job down the road.
Bryan: That is such a cool story. Thank you so much professor for sharing all of that. I really appreciate your time.